Big Data, Bigger Impact: Meet the NYSCF Data Science Team
NewsQuiz time! Can you tell the difference between these cells (besides their color)?
If you can’t, you’re not alone. If you think you can, you’re probably wrong (sorry). What makes these cells different (in a scientifically significant way) can’t be determined by the human eye. At NYSCF, we have millions of images of cells like this, and we need ways to tease apart their minute differences to understand how they are impacted by disease.
Disease research is producing data like never before – it’s big, it’s important, and parsing through it to draw conclusions is no easy task. Luckily, our talented team of data scientists help us do just this.
Hear more from these talented individuals about their new artificial intelligence (AI)-powered tools, why we’re photographing millions of cells, and what’s on the horizon for large-scale disease research.
Why is data science important for disease research, especially here at NYSCF?
“The norm in many academic labs or scientific companies is often that a lot of the work is done by hand,” noted Neeloy Bose (NYSCF Associate Scientist, Discovery Platform). “You’re working with maybe one plate at a time, constituting about a hundred thousand cells or so. But because of the automated capacities here at NYSCF, we can work with so many more plates with millions of cells in the same amount of time.”
Neeloy is referring to The NYSCF Global Stem Cell Array® – our automated platform for producing, analyzing, and running experiments on cells. The Array takes the typically artisanal process of cell production and hands it over to robots that create the standardized, high quality cells needed to do effective, large-scale research. And thanks to the Array, every day is picture day for NYSCF’s cells.
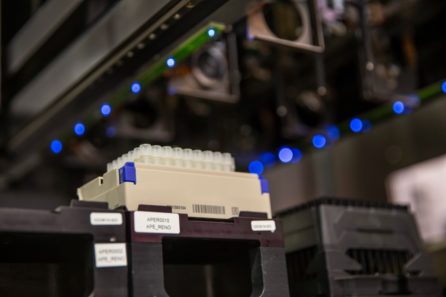
“I think Dan [Paull, NYSCF SVP of Discovery & Platform Development] said once that we have 200 plates in culture [running through the robotics] at a time, and every night, the machines take more than 200,000 measurements based on images of these cells,” added Jeff Winchell (Associate Data Scientist).
“Generating data in this semi-automatic way is very unique,” noted Gabriel Comolet (Associate Data Engineer). “It’s only possible with robotics like this.”
“Until I came here, I couldn’t even fathom working with so much data,” agreed Neeloy. “But when you work with smaller sample sizes, you can’t extract results the way we do. We’re at a point where we can create all this information, but we need these advanced data science tools to really make anything of it, and to further the race to cures.”
How are you using AI to create new tools?
The team’s newest tools center around imaging cells – essentially, taking photos of them to assess how they’re growing, as well as how they are impacted by disease.
“If we’re able to capture the differences between cells from healthy donors and diseased donors, then we can capture the root and the impact of the disease,” explained Bianca Migliori, PhD (Principal Data Scientist). “And we do that through imaging, because this allows us to gather a lot of information in a non-invasive way.”
Their first tool, called FocA – described in a recently published SLAS Discovery paper – helps manage quality control of cell images by determining whether they are in-focus. Just like you can’t judge a blurry photo of a person, you can’t judge a blurry photo of a cell either.
“We use these high-powered microscopes to record statistics about the cells we grow – and one thing we look at is image quality,” noted Bianca. “It’s important that our images are in-focus so we can use them to draw conclusions. That’s what FocA does – it is able to do this kind of quality control on a large scale, identifying in near-real time which images out of thousands are viable.”
While a person could perhaps tell whether an image is in-focus or not, FocA’s power is in its speed and precision.
“FocA can analyze thousands of images in the time it would take a person to even sift through one image,” noted Neeloy. “Which is important when you’re creating hundreds of thousands of images overnight.”
Their other tool – ScaleFEx – helps scientists cheat at that quiz we made you take at the beginning of this article, deciphering differences between cells, as well as which of these differences are most critical.
“ScaleFEx, on the other hand, helps us collect a lot of different features from cells that we can then use to try to distinguish between those that are healthy versus diseased,” said Bianca. “It’s a very fast and comprehensive tool that uses AI to understand which measurements are most important when we compare cells.”
Why is AI so important for your work?
“Both FocA and ScaleFEx are powered by AI, and the determinations they make wouldn’t be detectable to the human eye,” said Bianca. “In the case of ScaleFEx, only AI can dig deep enough to find what makes cells from different people different, as well as what makes them different from each other.”
Once again, the blessing (and curse) of having massive amounts of data also factors in here.
“A human person can try to go through all the data we produce, but it would take forever,” explained Bianca. “There’s just too much. Even if it was a task everyone could do, like telling cats and dogs apart, if you have millions and millions of images, having people look at each image and tell you if it’s a cat or a dog would take a ton of time. AI can do it very quickly. It saves scientists time and helps refine processes to make sure everything is running smoothly and accurately.”
What is next for integrating AI into NYSCF’s work?
The team has a few ideas rattling around in their brains. One has to do with generative AI – or AI that can produce content rather than just analyze it (think: ChatGPT).
“Maybe we could one day use generative AI to make predictions about how cells will look in the future, or under different conditions, rather than just how they look now,” postured Bianca. “Can we make an old cell look young, or a young cell look old? What would it look like if we treat it with a certain drug? AI could help us shift the same cell into different states. At this time, it’s a bit of a fantasy, but a few years ago we didn’t think ChatGPT would be possible, so we’ll see what the future brings.”
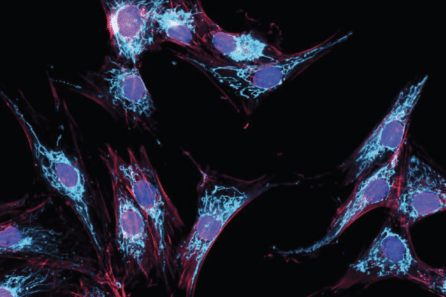
New tools could also continue assisting with quality control and analysis.
“I’m working on an AI-powered algorithm that could potentially pinpoint reasons why some batches of cells don’t pass quality control checks, so that we can maybe catch that before it happens,” added Jeff.
“And with ScaleFEx, we’re now looking to expand it to many different cell types,” said Bianca. “The initial version was relevant mostly to fibroblasts [cells found in connective tissue]. We now want to adapt it to neurons, organoids [3D aggregates of tissue made from stem cells], and other cells we use here for research.”
What is your favorite part about your job and working with this team?
“Working so closely with biologists and people with different backgrounds is especially cool because I learn so many different things,” said Jeff. “It’s very interdisciplinary. And even just within this team, we have different strengths, and I learn a lot from Bianca as a mentor.”
“I think my favorite part is troubleshooting,” shared Neeloy. “Obviously it’s nice when something runs perfectly, but I think the fun part is when something goes wrong because then it usually becomes a team effort that requires a lot of brain power. Those road bumps are gratifying because that’s when I learn the most, and that’s how we level up and do better science.”
“We might be a small team, but we do very different things, and if you don’t know something, you can go learn about it and interact with so many different experts,” added Gabriel. “That’s very exciting.”
“I love my team,” said Bianca. “Everyone is so driven and passionate about what they do, and we work together very well to provide important insights for our scientists. We all value each other and make sure we’re supportive. I’m very proud of all of them!”